Overall Research Goal
Our lab is driven by a central goal: to develop cutting-edge computational tools and integrative omics approaches that advance precision cancer medicine. We aim to extract actionable insights from complex biological datasets and translate them into hypotheses and models that improve patient care. Our work focuses on building robust platforms that unify proteomics, transcriptomics, genomics, and clinical data, while leveraging AI to drive hypothesis generation, biomarker discovery, and therapeutic modeling. Currently, our research spans three key areas: (1) the development of innovative bioinformatics tools, (2) cancer proteomics, and (3) functional genomics.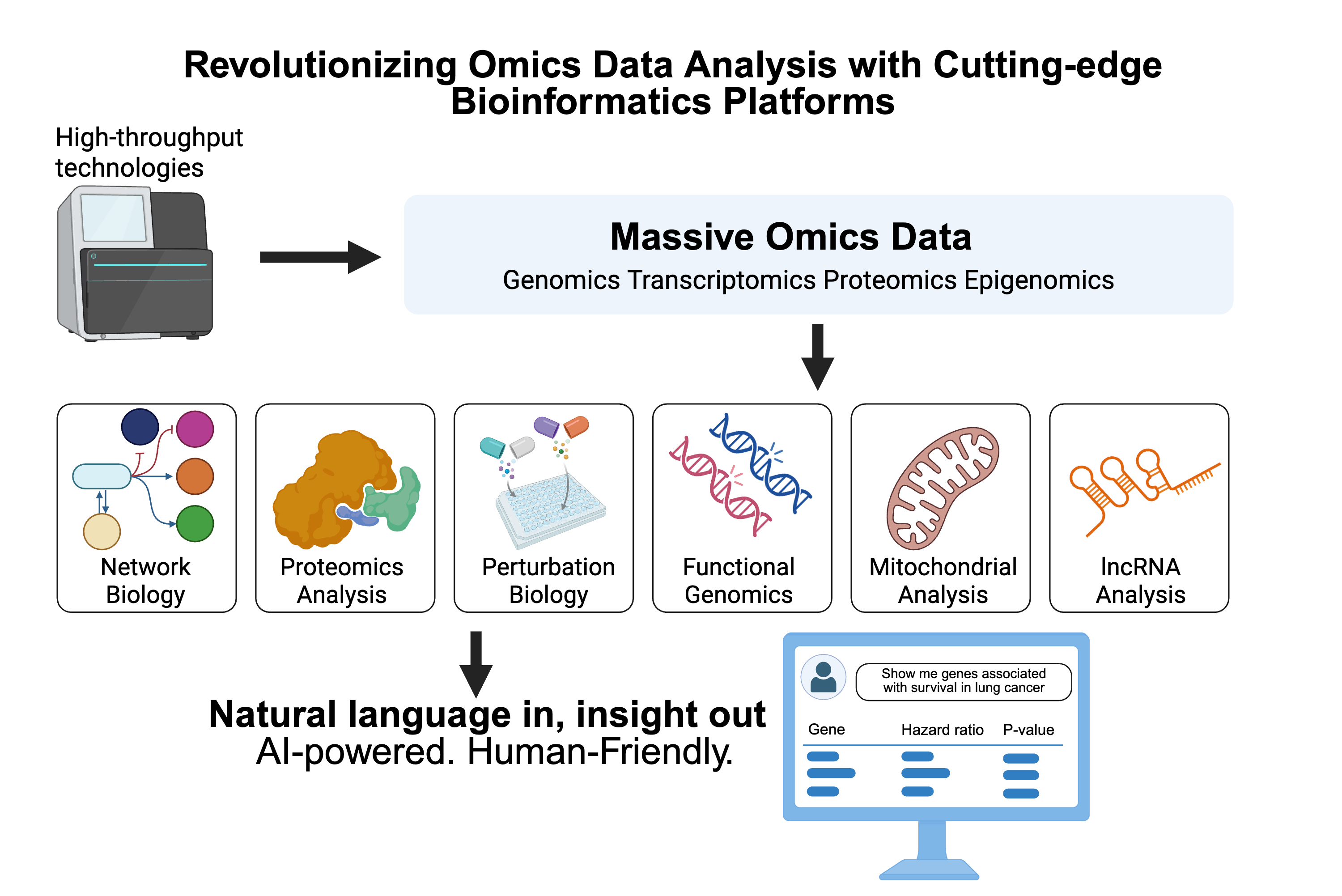
Revolutionizing Omics Data Analysis with Cutting-edge Bioinformatics Platforms
Over the last two decades, high-throughput molecular profiling technologies have revolutionized biomedical sciences. Various types of omics data generated from thousands of patients, animal models, and cell lines are accumulating rapidly, particularly through large consortium projects. These rich datasets have provided unprecedented opportunities to systematically characterize molecular mechanisms and develop related biomedical applications. However, the surge in data also presents a significant challenge for researchers aiming to extract meaningful insights through data analysis.
Over the past decade, we have pioneered the development of a range of state-of-the-art bioinformatics platforms tailored for complex cancer omics data analysis. These platforms span a wide array of specialized fields, including network biology through SurvNet (Nucleic Acids Research, 2012), proteomics via TCPA and MCLP (Nature Methods, 2013; Cancer Cell, 2017), perturbation biology with CPPA (Cancer Cell, 2020), functional genomics through FASMIC-mutation and FASMIC-fusion (Cancer Cell, 2018; Science Advances, 2022), mitochondrial analyses via TCMA (Nature Genetics, 2020), and long noncoding RNA research with TANRIC (Cancer Research, 2015). This suite of tools has achieved global reach, with over 200,000 users from more than 100 countries.
Building on this solid foundation, we have developed DrBioRight, a natural language-oriented, artificial intelligence (AI)-driven omics data analysis platform (Cancer Cell, 2021; Nature Communications, 2025). Unlike traditional bioinformatics tools, DrBioRight features a simple online chat interface with a single input and output area, enabling intuitive user interaction through natural language. This innovative approach is designed to usher in a new research paradigm—maximizing the utility of omics data, accelerating biomedical discovery, and ultimately leading to improved health outcomes for all.
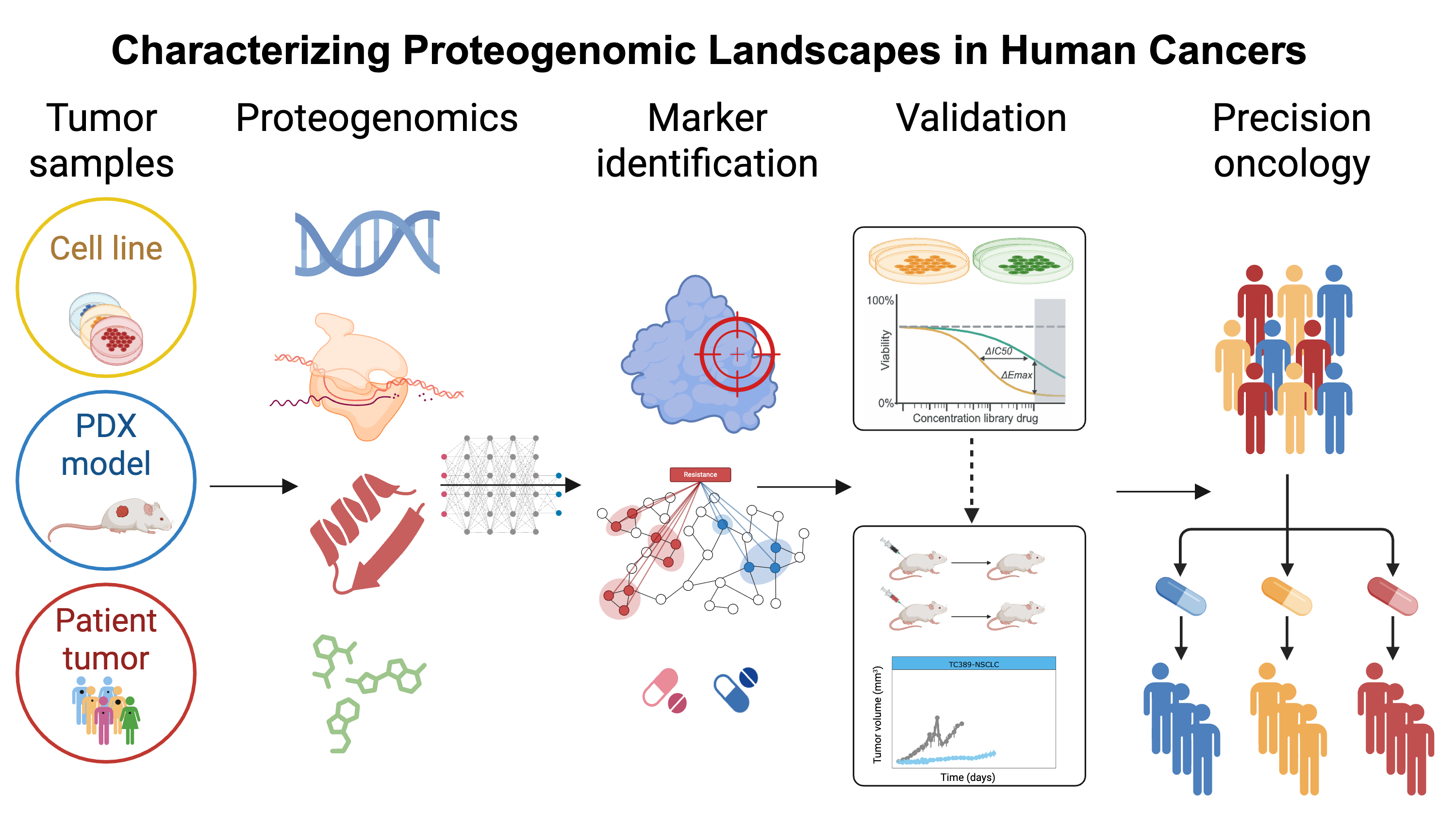
Characterizing Proteogenomic Landscapes in Human Cancers
Proteins, as the fundamental functional units of the cell and direct targets of most therapeutics, provide crucial insights into identifying suitable preclinical models and characterizing underlying disease mechanisms. Despite the abundance of DNA and RNA data, large-scale protein expression datasets remain scarce, particularly across diverse cancer types with substantial sample sizes. One of my primary research interests lies in exploring and analyzing proteomics data in conjunction with parallel multi-omics, clinical, and phenotypic datasets to formulate data-driven hypotheses. This multidisciplinary approach enables a comprehensive understanding of heterogeneous and complex data, facilitating the discovery of novel biomarkers and providing insights into cancer vulnerabilities and therapeutic strategies. For instance, through computational and machine learning methods, we demonstrated that cell line data can recapitulate mutation effects on protein expression observed in patient samples and that RPPA-based protein markers are powerful predictors of drug sensitivity (Li and Lu et al., Nature Methods, 2013; Li and Zhao et al., Cancer Cell, 2017). Building on this, we applied adaptive protein expression profiling and showed that perturbed protein responses significantly enhance the predictive accuracy for drug sensitivity, offering mechanistic insights into drug resistance.
We further developed a computational framework to predict effective drug combinations, which was supported by independent clinical evidence and experimental validation (Zhao and Li et al., Cancer Cell, 2020). Additionally, our systematic analyses revealed that protein expression profiles provide deep insights into inter-tumor heterogeneity, cancer dependencies, and act as sensitive indicators of the functional impact of somatic alterations. We also established a protein-centered strategy to identify synthetic lethality pairs, one of which was experimentally validated. Moreover, by analyzing metastatic potential across cell lines, we identified clinically relevant protein markers associated with metastasis. Collectively, our work represents a valuable contribution to advancing the understanding of cancer biology, identifying protein biomarkers, and developing novel therapeutic strategies (Li et al., Nature Cancer, 2024).
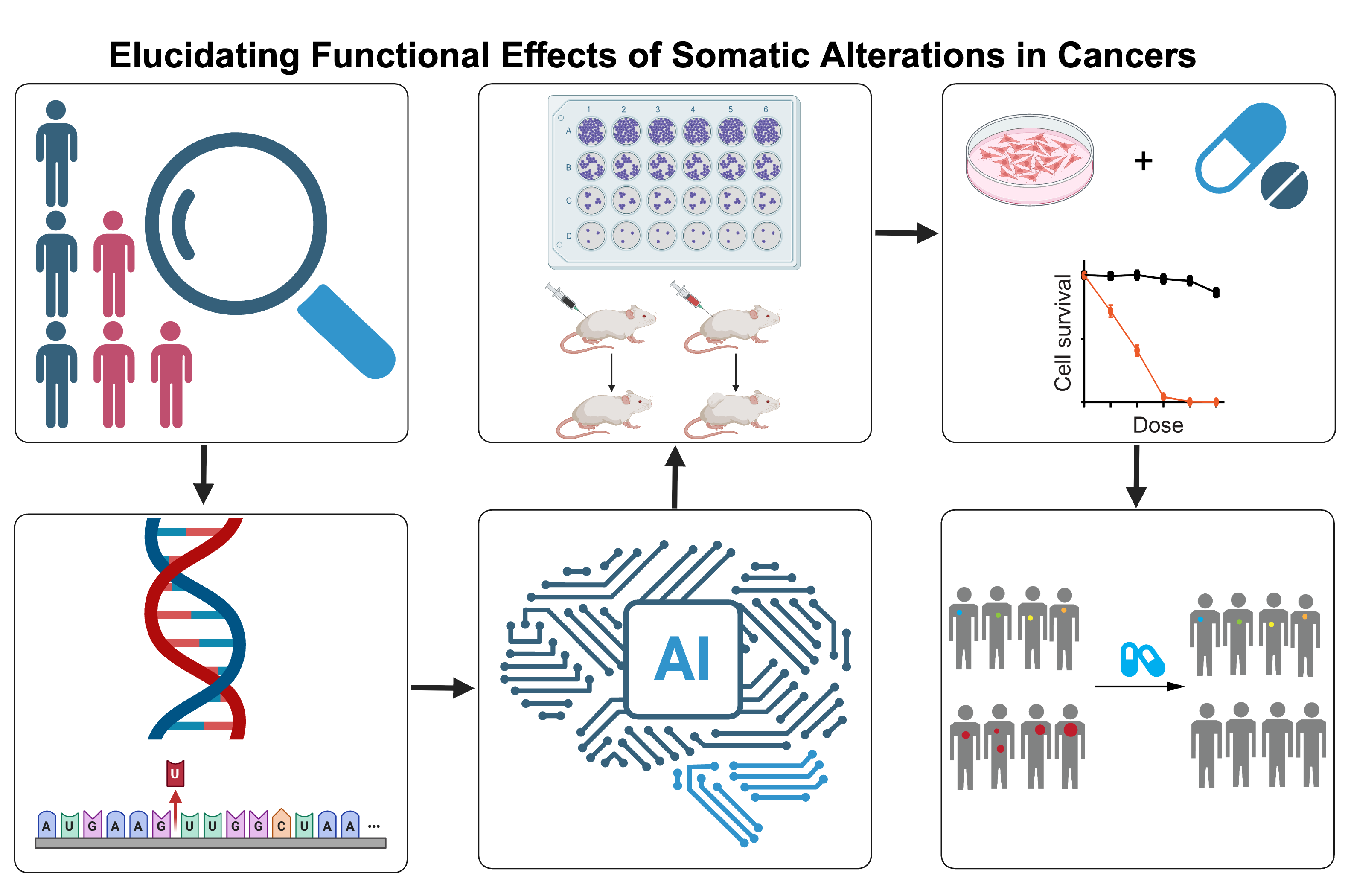
Elucidating Functional Effects of Somatic Alterations in Cancers
Next-generation sequencing technologies, including consortium projects such as TCGA, have identified thousands of unique mutations and fusions across cancer types. Such alterations observed in cancer tissues may exert different functional effects, ranging from oncogenic activation to tumor suppression or having no obvious functional impact. Importantly, diverse mutations within the same gene are often observed, depending on the tumor context. A critical challenge in developing and implementing cancer therapies and improving patient care is distinguishing causal driver mutations from non-pathogenic passenger variants and elucidating their oncogenic mechanisms. Thus, there is an urgent need to systematically characterizing large numbers of cancer variants of unknown significance (VUS).
Using a versatile and sensitive functional genomic approach, we systematically assessed the effects of more than 1,000 somatic alterations on cell viability and downstream signaling (Ng and Li, et al., Cancer Cell, 2018). Specifically, using this dataset as a benchmark, we have computationally identified and annotated mutations from patient cohorts and assessed their functional effects using cutting-edge prediction algorithms. By incorporating functional proteomic profiling, we conducted integrative analyses to elucidate the functional impacts of driver mutations on pathway activities. Additionally, our studies focusing on fusions have revealed that a significant fraction of low-frequency, or even unique, gene fusions possess functional effects and potential therapeutic relevance, which has important implications for patient management (Li et al., Science Advances, 2022). In summary, our functional genomic approach represents an initial but critical attempt to improve the clinical impact of targeting rare gene fusion events.